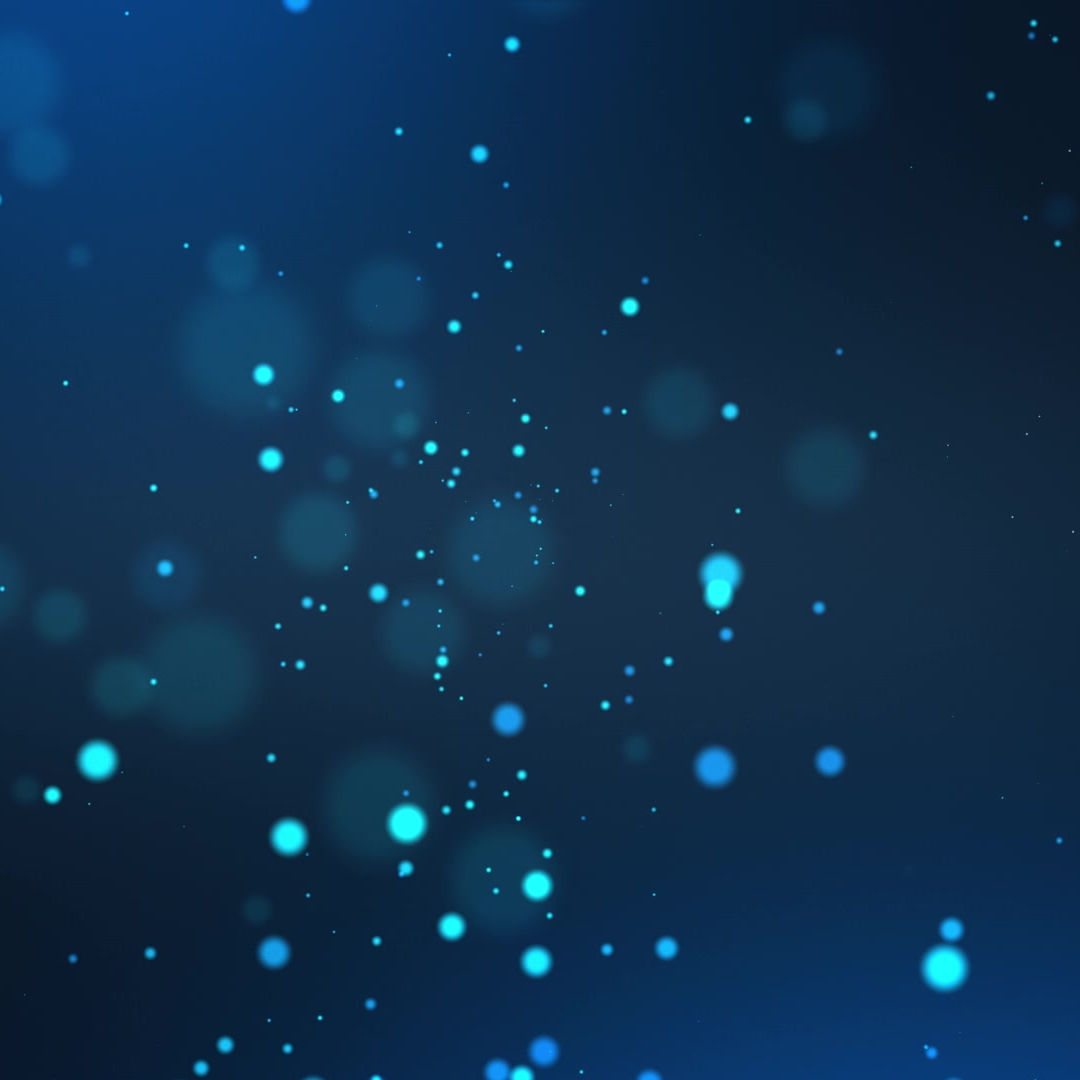
Particle Physicist
Lecturer in Computational Physics
Department of Physics and Astronomy
University of Manchester, UK
LATEST PROJECTS
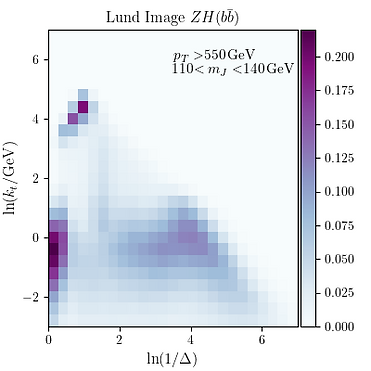
Higgs tagging with the Lund jet plane
We present a procedure to separate boosted Higgs bosons decaying into hadrons, from the background due to strong interactions. We employ the Lund jet plane to obtain a theoretically well-motivated representation of the jets of interest and we use the resulting images as the input to a convolutional neural network. In particular, we consider two different decay modes of the Higgs boson, namely into a pair of bottom quarks or into light jets, against the respective backgrounds. The performance of the tagger is compared to what is achieved using a traditional single-variable analysis which exploits a QCD inspired color-singlet tagger, namely the jet color ring observable. See arxiv: 2105.03989 for more details.
Model-independent new physics searches using machine learning (ML)
Anomaly Awareness: We proposed a new algorithm for anomaly detection called Anomaly Awareness, which learns about normal SM events while being made aware of the BSM anomalies. We showed how it works in a well-known fat jet topology for new physics searches and tested it for a few BSM scenarios: EFT Higgs, (leading) fat jet from resonance decay with multiple subsets. It proved effective on BSM anomalies not seen before, and became more robust after becoming aware of a range of anomalies. Our method requires at least two categories of SM events, therefore, by building a set of binary classifiers for the SM processes we can use it on the LHC data. For more details see arXiv:2007.14462 [cs.LG].
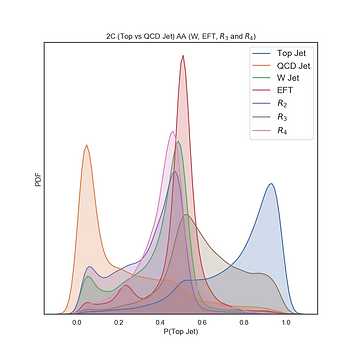
ML for dark matter searches

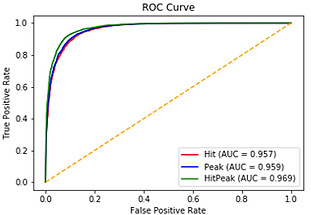

For the collider DM searches, we demonstrated the performance of ML methods to disentangle different DM signals (WIMP DM candidate in the form of a SUSY neutralino.} considering monojet (and MET) channel. For the direct DM searches at Xenon1T experiment, we suggested the use of Convolutional NNs to enhance the ability to distinguish a WIMP from background events using the morphology of hit patterns in the time projection chamber. See arxiv: 1911.09210 and arxiv:1910.06058 for more details.
SMEFT study using ML
We used ML in the SMEFT framework, in order to capitalise on the kinematic information in the ``Higgs boson associated production with a massive vector boson'' (VH channel). We found that the use of a shallow neural network (with Asimov Loss Function) dramatically increases the sensitivity to deviations in VH respect to previous estimates. Following the strategy of this work, we are performing a global analysis for the new physics deformations in the Effective Field Theory framework. For full details see arxiv: 1902.05803
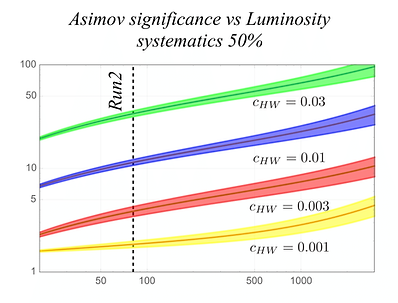